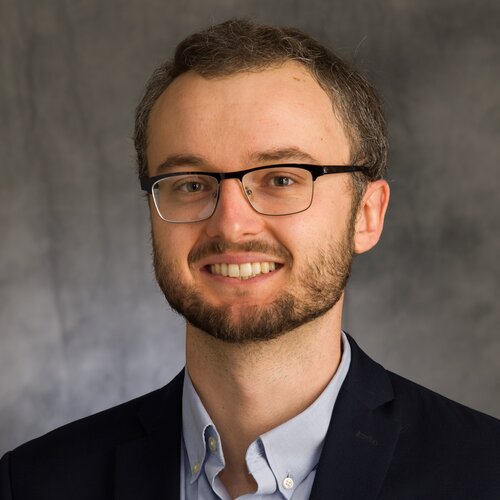
Contact Information
myerscou@illinois.edu
(217) 300-4463
Department of Chemistry
350E Noyes Lab
600 S. Mathews Avenue
MC-712
Urbana, IL 61801
Research Areas
Additional Campus Affiliations
Affiliate faculty member, Chemical and Biomolecular Engineering
Affiliate faculty member, Materials Science and Engineering
Affiliate faculty member, Beckman Institute - AI for Materials
Biography
Prof. Jackson obtained his B.A. in Physics from Wesleyan University in 2011, followed by a Ph.D. in Chemistry in 2016 working with Prof. Mark Ratner and Prof. Lin Chen at Northwestern University. He was subsequently a Named Fellow in the Center for Molecular Engineering at Argonne National Laboratory and the University of Chicago, supervised by Prof. Juan de Pablo. Prof. Jackson was promoted to an Assistant Scientist in 2019, and joined the faculty at UIUC in January 2021.
Research Interests
Coarse-graining, multiscale simulations, electronic processes, soft materials, machine learning
Research Description
The Jackson Lab understands and designs electronic processes in soft materials. We are interested in soft materials that exhibit useful semiconducting, energy storage, energy generation, bioelectronic, or degradable functionality. There is a strong effort devoted to multiscale computational method developments that enable electronic predictions at the mesoscale.
Projects include:
Electronic Coarse-Graining
Molecular quantum mechanics has advanced to the point that high-accuracy quantum mechanical observables may be computed for arbitrary chemical structures. However, to fully account for morphological heterogeneity of soft materials (e.g. organic molecules, polymers), one must simulate system sizes on the order of 10-100 nm. This length scale is only accessible via computationally efficient classical simulations using reduced resolution (coarse-grained) models – in these models one cannot solve the Schrödinger equation directly because the atomic degrees of freedom have been averaged into effective coarse-grained particles. Our research goal is to develop efficient and accurate electronic prediction models that do not require the specification of all atomic degrees of freedom. These models will provide access to optical, electronic, and reactive predictions for soft materials at the mesoscopic length scales relevant to real devices of critical use in semiconducting, bioelectronic, and mechanochemistry applications.
Electron and Ion Conduction in Organic Materials
Semiconducting molecules and polymers combine the mechanical and thermophysical advantages of soft materials with optoelectronic functionality common to inorganic semiconductors (e.g. Silicon, GaAs). In recent years, the application of these materials classes in biological systems as signal transducers at neural interfaces has emerged. To design materials for these systems requires the challenging multiscale prediction of morphological structure, electronic conductivity, and ionic conductivity. Our research goal is to develop computational models capable of efficiently describing the strong coupling between electronic, ionic, and morphological degrees of freedom in organic materials and to use these models to understand the fundamental tradeoffs between electronic and ionic conductivity in polymeric materials.
AI-Guided Soft Materials Design
A crucial challenge for soft materials design is the strong coupling of disparate length and timescales that mediates materials behavior; molecular degradation is influenced by temperature and humidity; electronic and ionic transport are controlled by mesoscale morphology; biological functionality is inherently hierarchical. This confluence of length and time scales requires the integration of a collection of theoretical, computational, and data-centric techniques to fully characterize material performance. In the past decade, machine-learning (ML) approaches to molecular modeling have transformed the landscape of materials research and engineering. Our research goal is to develop automated and synthetically-aware machine learning models that transform experimental design and closed-loop discovery efforts for soft materials. Specific directions in the group focus in two directions: (i) method development of generative machine learning models of polymers incorporating synthetic awareness (collaboration with IBM), (ii) closed-loop Bayesian optimization of photostable organic molecules (collaboration with Molecule Maker Laboratory Institute).
Awards and Honors
School of Chemical Sciences Faculty Teaching Award
ACS OpenEye/Cadence Outstanding Junior Faculty Award in Computational Chemistry
Early Career Board, Journal of Chemical Theory and Computation
Research Corporation Scialog Fellow for Automating Chemical Laboratories
Department of Energy Early Career Program Award
Lincoln Excellence for Assistant Professor (LEAP) Scholar, UIUC LAS
Dreyfus Machine Learning in the Chemical Sciences and Engineering Award
ACS Petroleum Research Fund Doctoral New Investigator Award
3M Nontenured Faculty Award